Spotlight on Control Group Testing: the good, the bad and the not-so-pretty
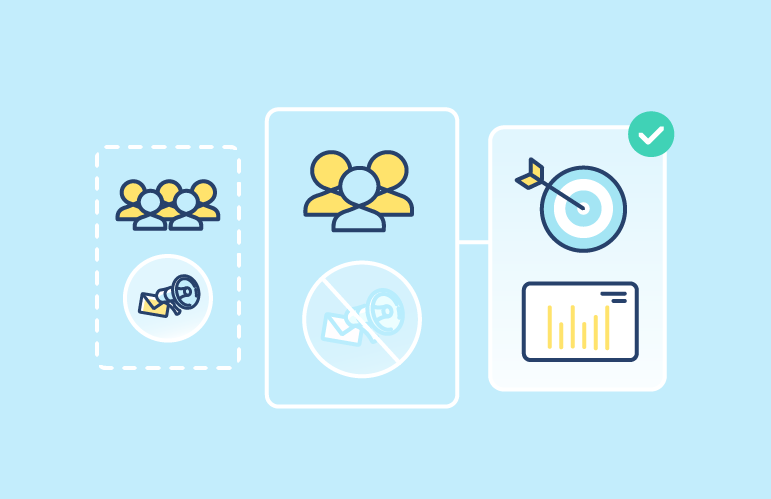
Catégorie : Stratégie CRM
As today’s evolving technology takes digital CRM to complex new heights, control group testing has remained a reassuring go-to for gauging how innovative targeting solutions measure up. But as we’ll explore here, this good old-fashioned practice is not always a marketer’s best friend.
As a Data Product Manager at Splio, I spend a lot of time analyzing campaign performance for long-term engaged customers. I’ve done more than my share of control group testing, as a way of quantifying the added value Splio’s AI-first targeting solution brings to our clients.
Control group testing can be a valuable tool, providing more reliable results than other attribution methods, but it’s important to understand its limits. Having practiced and studied it from every angle for a couple of years now, I’d like to share some of my insights on the subject.
First though, let’s back up a minute…what is control group testing again?
Basically, it involves isolating or “freezing” part of a customer base (typically 10%), leaving them out of every campaign you want to test, then measuring the performance delta between the control group and the test group. This allows you to measure the performance of all the campaigns tested, everything else being equal.
A number of things can be evaluated using this method: additional campaign revenues generated by the test group, additional number of orders or buyers, website clicks, etc. Of course, what control group testing can’t do is evaluate campaigns where you don’t know exactly which customers have been reached (TV ads, subway posters…) or where revenue can’t be measured on a per-customer basis.
I’ll say it again: significance and certainty are KEY. What do I mean by “significance”? Let’s say you use control group testing for a campaign, and the test group results show an additional 100 sales out of 1000 total. That’s probably a large enough percentage to be considered significant. But 100 out of 10 million? Not so much.
The important thing here is determining whether results are reliable beyond the “statistical noise” – that is, the random element of consumer behavior and customers’ arbitrary distribution between groups.
By “certainty” I mean the reliability of results being measured, which is not always easy to determine. But the whole point of control group testing is its accuracy vs. other attribution methods, sooo…its value is only as good as its degree of certainty, right?
Statistical significance can be illustrated using a range of results at a given certainty level, for example: 95% certainty that additional number of sales will be between 75-125. Statistically, this means there are 19 chances in 20 that additional number of sales will be within this range. Unfortunately, the narrower the range, the lower the certainty.
Graph showing the incrementality uncertainty curve for control group testing:
Bearing all that in mind, let’s take a look at some of the common perils and pitfalls of control group testing…
For campaigns where your base includes disproportionately “big” buyers (professionals buying bulk, etc.), testing results can be skewed in one direction or another – and you won’t know how. Case in point: for one of our clients, their top 100 customers account for over 10 million euros in annual revenue…which is about the same order of magnitude as the additional revenue generated by Splio.
Since it’s impossible to control the distribution of these “outliers” between test groups, the only solution is to eliminate them from testing, in order to avoid throwing off the results.
Whatever the campaign, reliable results depend on a significant (there’s that word again) number of sales. Sometimes there’s confusion about just what constitutes sufficient volume, so for the record: 30 sales is not enough.
For testing results to be reliable, number of sales should be in the hundreds at least. The same principle applies to email volume: low mailing volume = low testing reliability. This is true for retailers of all sizes, including big retailers with high overall revenue but a relatively small number of sales and emails sent – like one of our clients, who markets high price-tag travel packages.
It’s understandable: marketers want to know which of their campaigns generate extra revenue, so testing them individually is an enticing pursuit. This is a slippery slope though, as the results for individual campaigns just aren’t meaningful most of the time.First of all, single campaigns have a relatively slight impact on sales – too slight to cross the threshold of (wait for it…) significance.
Also, some marketers make the mistake of using different control groups from one campaign to the next, which makes comparing results between campaigns even less meaningful. It’s important to take a long-term, “big picture” approach; by testing over time, in a consistent way, you’ll get a much more reliable perspective in the end.
Customer fatigue management is another factor that can distort the results of control group testing. Especially for big retailers with high mailing volume, who rely heavily on these planning mechanisms to avoid agenda conflicts. Splio’s own pressure management system takes control groups into account, but many retailers’ systems aren’t as sophisticated.
In cases where your planning tool filters out part of the test group base, the control group ends up being “over-solicited” in comparison. This imbalance can be even stronger with call-to-action campaigns – those special mailings sent out to a selection of top customers, who may wind up over-represented in the control group.
Despite the allure of control group testing, in most cases…you probably shouldn’t try this at home, folks. It’s a complex undertaking, and meaningful results are very hard to come by. Also, let’s not forget that it comes at a cost: by freezing a significant portion of your customer base, you’re depriving yourself of the potential additional revenue from these users.
At Splio, we stand behind the merits of our Deep Learning technology, but we’re not alone; our solution has been tried and tested 6 ways to Sunday, by data scientists from e-commerce retailers around the world. Hundreds of tests have confirmed that our targeting solution leads to significantly higher performance when it comes to campaign revenues.
But there’s more: Splio enables marketers to orchestrate complex campaign schedules, an additional advantage that is non-negligible. Splio’s clients value our solution not only for the quantifiable results it provides, but because it brings real campaign intelligence to their marketing, and optimizes their entire campaign strategy – benefits that are harder to measure, but every bit as certain and significant.
Sommaire
À lire aussi